Anand D. Sarwate
Associate Professor of ECE, Rutgers University, anand.sarwate@rutgers.edu
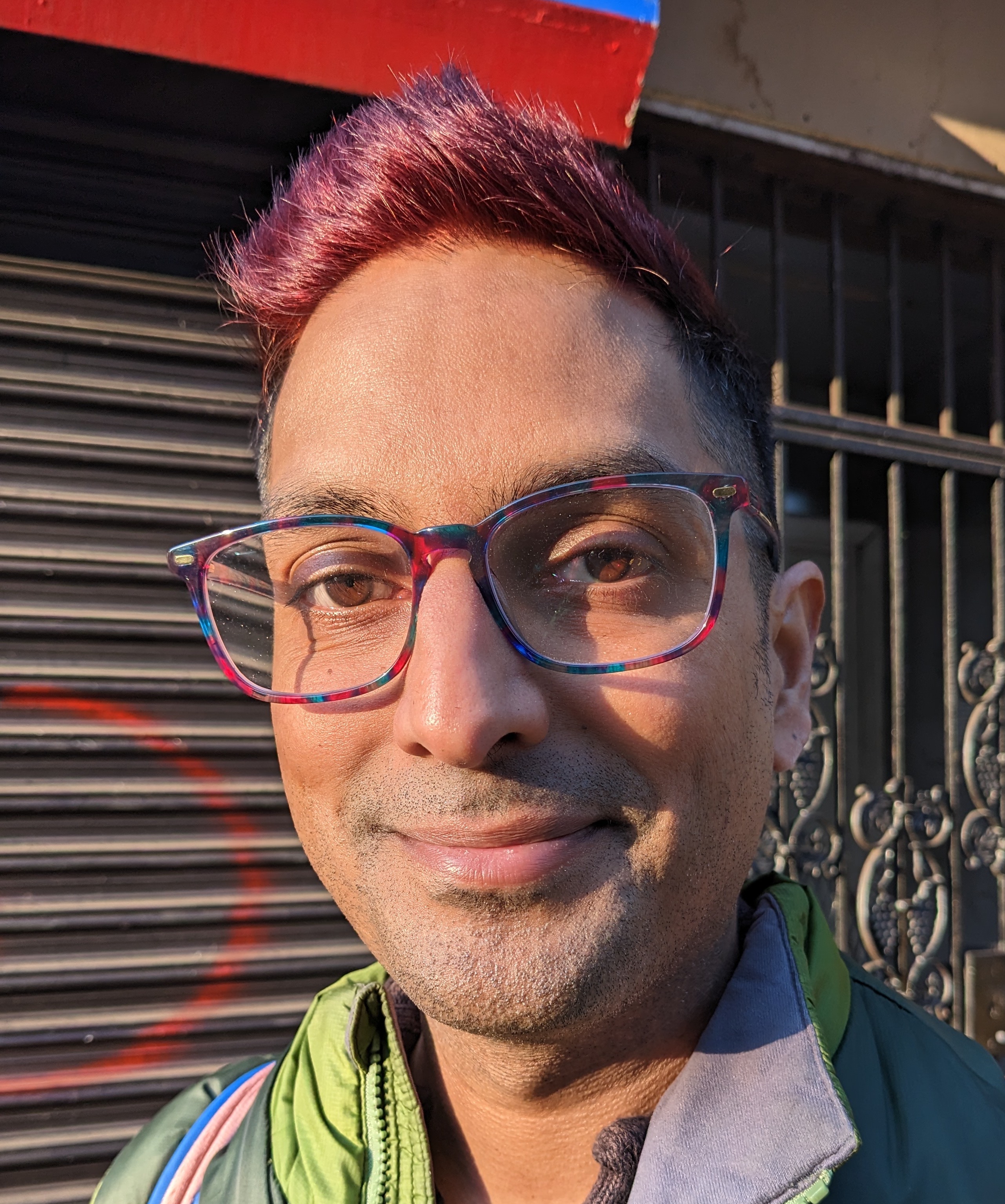
CoRE Building Rm. 517
Busch Campus
Rutgers University
Piscataway, NJ 08854-8058
(848) 445-8516
I am an Associate Professor in the Department of Electrical and Computer Engineering
at Rutgers, The State University of New Jersey.
I also am a member of the graduate faculty in the Department of Computer Science
and the Department of Statistics.
I like to work on problems that involve probability, mathematical statistics,
and optimization, with applications in information theory, communication,
signal processing, and machine learning. I’m particularly interested in
how these things intersect in the context of distributed/decentralized
systems with constraints like privacy, bandwidth, latency, power, and so on.
This page is still somewhat under construction. I anticipate that will be the state for quite a while.
news
Jan 19, 2024 |
To appear at ICLR 2024 (spotlight): A. W. Engel, Z. Wang, N. Frank, I. Dumitriu, S. Choudhury, A. Sarwate, T. Chiang, Faithful and Efficient Explanations for Neural Networks via Neural Tangent Kernel Surrogate Models. To appear at ICASSP 2024: J. Hoyos Sanchez, B. Taki,, W. U. Bajwa, A. D. Sarwate, Federated Learning of Tensor Generalized Linear Models with Low Separation Rank. I’m teaching ECE 549 this semester, which was traditionally called Detection and Estimation Theory. |
---|---|
Nov 27, 2023 | I have been appointed as a Distinguished Lecturer for 2024-2025 by the IEEE Information Theory Society. I’m happy to visit to give a talk! |
Nov 22, 2023 | I received the Outstanding Engineering Professor Award from the Rutgers School of Engineering! |
Sep 22, 2023 |
Some recent activity:
|
Jun 20, 2023 |
A few new papers to appear:
|
selected publications
- S. Li, P. Krishan, S. Jaggi, M. Langberg, and A. D. Sarwate, “Computationally Efficient Codes for Adversarial Binary-Erasure Channels,” in Proceedings of the 2023 IEEE International Symposium on Information Theory (ISIT), 2023, pp. 228–233.
- R. Islam, K. N. Keya, S. Pan, A. D. Sarwate, and J. R. Foulds, “Differential Fairness: An Intersectional Framework for Fair AI,” Entropy, vol. 25, no. 4, Apr. 2023.
- H. Gazula, K. Rootes-Murdy, B. Holla, S. Basodi, Z. Zhang, E. Verner, R. Kelly, P. Murthy, A. Chakrabarti, D. Basu, S. Bhagyalakshmi Nanjayya, R. Lenin Singh, R. Lourembam Singh, K. Kalyanram, K. Kartik, K. Kalyanaraman, K. Ghattu, R. Kuriyan, S. S. Kurpad, G. J. Barker, R. D. Bharath, S. Desrivieres, M. Purushottam, D. P. Orfanos, E. Sharma, M. Hickman, M. Toledano, N. Vaidya, T. Banaschewski, A. L. W. Bokde, H. Flor, A. Grigis, H. Garavan, P. Gowland, A. Heinz, R. Brühl, J.-L. Martinot, M.-L. Paillére Martinot, E. Artiges, F. Nees, T. Paus, L. Poustka, J. H. Fröhner, L. Robinson, M. N. Smolka, H. Walter, J. Winterer, R. Whelan, J. A. Turner, A. D. Sarwate, et al., “Federated Analysis in COINSTAC Reveals Functional Network Connectivity and Spectral Links to Smoking and Alcohol Consumption in Nearly 2,000 Adolescent Brains,” Neuroinformatics, vol. 21, pp. 287–301, Apr. 2023.
- Z. Wang, A. Engel, A. Sarwate, I. Dumitriu, and T. Chiang, “Spectral evolution and invariance in linear-width neural networks,” no. arXiv:2211.06506 [cs.LG], ArXiV, Nov-2022.
- Y. Zhang, S. Vatedka, S. Jaggi, and A. D. Sarwate, “Quadratically Constrained Myopic Adversarial Channels,” IEEE Transactions on Information Theory, vol. 68, pp. 4901–4948, Aug. 2022.
- Y. Zhang, S. Jaggi, M. Langberg, and A. D. Sarwate, “The Capacity of Causal Adversarial Channels,” in Proceedings of the 2022 IEEE International Symposium on Information Theory (ISIT), 2022.
- N. Sathyavageeswaran, R. D. Yates, A. D. Sarwate, and N. Mandayam, “Privacy Leakage in Discrete Time Updating Systems,” in Proceedings of the 2022 IEEE International Symposium on Information Theory (ISIT), 2022.